Deep Learning Patterns and Practices
- 13h 45m 3s
- Andrew Ferlitsch
- Manning Publications
- 2021
Discover best practices, reproducible architectures, and design patterns to help guide deep learning models from the lab into production.
In Deep Learning Patterns and Practices you will learn:
- Internal functioning of modern convolutional neural networks
- Procedural reuse design pattern for CNN architectures
- Models for mobile and IoT devices
- Assembling large-scale model deployments
- Optimizing hyperparameter tuning
- Migrating a model to a production environment
The big challenge of deep learning lies in taking cutting-edge technologies from R&D labs through to production. Deep Learning Patterns and Practices is here to help. This unique guide lays out the latest deep learning insights from author Andrew Ferlitsch’s work with Google Cloud AI. In it, you'll find deep learning models presented in a unique new way: As extendable design patterns you can easily plug-and-play into your software projects. Each valuable technique is presented in a way that's easy to understand.
About the technology:
Discover best practices, design patterns, and reproducible architectures that will guide your deep learning projects from the lab into production. This awesome audiobook collects and illuminates the most relevant insights from a decade of real world deep learning experience.
About the audiobook:
Deep Learning Patterns and Practices is a deep dive into building successful deep learning applications. You’ll save hours of trial-and-error by applying proven patterns and practices to your own projects. Tested code samples, real-world examples, and a brilliant narrative style make even complex concepts simple and engaging. Along the way, you’ll get tips for deploying, testing, and maintaining your projects.
About the audience:
For machine learning engineers familiar with Python and deep learning.
About the Author
Andrew Ferlitsch is an expert on computer vision, deep learning, and operationalizing ML in production at Google Cloud AI Developer Relations.
In this Audiobook
-
Chapter 1 - Designing modern machine learning
-
Chapter 2 - Deep neural networks
-
Chapter 3 - Convolutional and residual neural networks
-
Chapter 4 - Training fundamentals
-
Chapter 5 - Procedural design pattern
-
Chapter 6 - Wide convolutional neural networks
-
Chapter 7 - Alternative connectivity patterns
-
Chapter 8 - Mobile convolutional neural networks
-
Chapter 9 - Autoencoders
-
Chapter 10 - Hyperparameter tuning
-
Chapter 11 - Transfer learning
-
Chapter 12 - Data distributions
-
Chapter 13 - Data pipeline
-
Chapter 14 - Training and deployment pipeline
YOU MIGHT ALSO LIKE
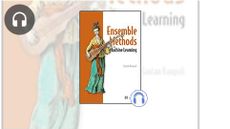